In today’s data-driven world, understanding the aspects of a data and analytics framework is crucial for businesses aiming to harness the power of information. This framework serves as the backbone for effective data management, guiding organizations in collecting, analyzing, and interpreting data to drive strategic decisions.
From data governance to analytics methodologies, each component plays a vital role in transforming raw data into actionable insights. By grasping these key aspects, companies can enhance their operational efficiency and gain a competitive edge in their respective markets. Exploring this framework opens the door to unlocking the full potential of data analytics.
What Are the Aspects of the Data and Analytics Framework
A data and analytics framework encompasses several components that contribute to effective data management and utilization. These components include data governance, data architecture, data quality, analytics methodologies, and technology infrastructure.
Data Governance
Data governance establishes policies and standards that manage data integrity, security, and usability. It ensures compliance with regulations and ethical guidelines. Strong data governance enables organizations to maintain data accuracy and fosters trust among stakeholders.
Data Architecture
Data architecture defines the structure and organization of data across systems. It outlines how data is collected, stored, and accessed. A well-designed data architecture supports seamless integration of various data sources, facilitating efficient data analysis.
Data Quality
Data quality focuses on the accuracy, completeness, and reliability of data. High-quality data is essential for making informed decisions. Organizations implement data quality assessment and improvement processes to eliminate inconsistencies and ensure data reliability.
Analytics Methodologies
Analytics methodologies refer to techniques and processes used to analyze data. Common methodologies include descriptive, diagnostic, predictive, and prescriptive analytics. Each approach serves a different purpose, from understanding past performance to forecasting future trends.
Technology Infrastructure
Technology infrastructure involves the hardware and software systems that support data storage and analytics. Robust infrastructure allows for efficient data processing and analysis. Cloud computing, databases, and data visualization tools play key roles in enabling analytics capabilities.
The data and analytics framework integrates these components, promoting effective data practices and supporting organizations in leveraging data for strategic decision-making.
Key Aspects of the Data and Analytics Framework
The data and analytics framework consists of several key aspects that work together to facilitate effective data management and insightful decision-making. Each component plays a crucial role in the overall effectiveness of the framework.
Data Collection
Data collection involves the systematic gathering of relevant information from various sources. Organizations utilize methods such as surveys, sensors, and transactional systems to obtain both quantitative and qualitative data. By implementing standardized procedures, organizations ensure the consistency, reliability, and timeliness of the collected data. Effective data collection forms the foundation of a successful analytics strategy.
Data Storage
Data storage refers to the methods and systems used to retain collected data securely. Organizations employ databases, data lakes, and cloud storage solutions to manage large volumes of information. Considerations for data storage include accessibility, scalability, and data security. Properly structured storage enables efficient retrieval and enhances the overall performance of data retrieval applications.
Data Processing
Data processing transforms raw data into a usable format through processes such as cleaning, aggregating, and enriching data. Techniques like data transformation and integration ensure the information is consistent and compatible across various systems. Organizations leverage ETL (Extract, Transform, Load) processes to streamline and optimize data processing. This crucial step prepares data for further analysis.
Data Analysis
Data analysis involves examining and interpreting processed data to extract actionable insights. Organizations apply various analytical methodologies, including descriptive, predictive, and prescriptive analytics, to identify trends and make informed decisions. Advanced techniques such as machine learning and AI drive deeper insights, enabling organizations to predict future outcomes and evaluate potential strategies. Data analysis ultimately informs business strategies and supports data-driven decision-making.
Importance of Each Aspect
Each component of the data and analytics framework plays a vital role in ensuring that organizations leverage data effectively.
- Data Governance: Data governance establishes frameworks for maintaining data integrity and compliance, ensuring that organizations adhere to regulations and standards. It enables trust in data, allowing teams to make informed decisions backed by accurate information.
- Data Architecture: Data architecture delineates how data is structured and organized within systems. It facilitates efficient data management, allowing for streamlined access and integration, which is essential for robust analytics and quick decision-making.
- Data Quality: Data quality focuses on maintaining high standards of accuracy and reliability. Ensuring data quality reduces errors and enhances the dependability of insights generated, directly impacting business outcomes and strategic initiatives.
- Analytics Methodologies: Analytics methodologies encompass various techniques and tools used for data analysis. They enable organizations to identify trends, patterns, and correlations within data, leading to actionable insights that drive innovation and growth.
- Technology Infrastructure: Technology infrastructure encompasses the hardware and software systems supporting data processing. A scalable and reliable infrastructure ensures efficient data handling, enabling real-time analytics and better resource allocation.
- Data Collection: Data collection involves systematic gathering of information to ensure consistency and reliability. Efficient data collection processes capture diverse data types, enriching the datasets available for comprehensive analysis.
- Data Storage: Data storage solutions protect and retain data securely. Various approaches, including databases and cloud solutions, provide organizations with scalable options for data retention, ensuring easy access and retrieval when needed.
- Data Processing: Data processing converts raw data into usable formats. Through cleaning and integration, this aspect prepares data for analysis, allowing organizations to derive insights from large datasets efficiently.
- Data Analysis: Data analysis examines processed data to extract actionable insights. Utilizing advanced techniques, such as machine learning and AI, enhances predictive analytics capabilities, equipping organizations to adapt swiftly to changing market dynamics.
Challenges in Implementing the Framework
Organizations face several challenges during the implementation of a data and analytics framework. Understanding these hurdles facilitates better preparedness and strategic planning.
- Data Silos
Data silos occur when departments or teams operate independently without sharing data. This fragmentation limits access to comprehensive insights and hampers collaboration across the organization. - Data Quality Issues
Inconsistent data quality affects decision-making processes. Organizations often struggle with inaccuracies, duplications, and outdated information that hinder reliable analytics. - Change Management Resistance
Resistance to change presents a significant barrier. Employees may be reluctant to adopt new tools and processes, resulting in inadequate utilization of the framework and missed opportunities for improved efficiency. - Skill Gaps
Skill gaps in data literacy impact the successful execution of the framework. A lack of trained personnel capable of interpreting data and applying analytics methodologies can obstruct the organization’s progress. - Integration Challenges
Integrating diverse data sources and systems poses a challenge. Technical limitations often complicate the seamless connection of various tools and databases, leading to inefficiencies in data processing. - Compliance and Governance Risks
Navigating regulatory requirements is essential yet complex. Organizations must ensure compliance with data protection laws while implementing governance policies that maintain data integrity. - Resource Allocation
Inadequate resource allocation can hinder implementation. Limited budgets and personnel may restrict investment in necessary technology infrastructure and training needed for effective framework utilization. - Evolving Technologies
Rapid advancements in technology create challenges in keeping up. Staying current with emerging analytical tools and methodologies is crucial for organizations to remain competitive in a data-driven environment.
Addressing these challenges through strategic planning and investment promotes successful implementation and maximizes the benefits of the data and analytics framework.
Future Trends in Data and Analytics
Data and analytics continue to evolve rapidly, influencing organizational strategies and outcomes. Several key trends shape the future landscape of this domain.
1. Increased Use of Artificial Intelligence and Machine Learning
Artificial intelligence (AI) and machine learning (ML) enhance data analysis capabilities. Organizations leverage predictive models and algorithms to identify patterns, enabling proactive decision-making.
2. Real-Time Analytics
Real-time analytics transforms how businesses operate. Instant insights from streaming data allow organizations to react to market changes, customer behaviors, and operational inefficiencies quickly. For instance, the ability to stream data from MongoDB to BigQuery enables seamless data flow, facilitating real-time analytics across different platforms.
3. Data Democratization
Data democratization promotes access to data across all levels of an organization. By equipping employees with user-friendly analytics tools, businesses foster a data-driven culture where insights inform everyday activities.
4. Enhanced Data Privacy Regulations
Data privacy and protection regulations evolve regularly. Organizations prepare to comply with guidelines, emphasizing safe data practices and transparency to build consumer trust.
5. Growth of Edge Computing
Edge computing shifts data processing closer to the source. This minimizes latency and improves the performance of data-driven applications, especially in sectors like IoT and real-time analytics.
6. Focus on Data Quality Management
Data quality management remains critical. Organizations implement robust data governance frameworks to ensure accurate, reliable datasets support sound decision-making.
7. Integration of Cloud-Based Solutions
Cloud-based data storage and analytics solutions offer scalability and flexibility. Organizations transition to cloud environments to manage vast datasets and utilize advanced analytics services.
8. Collaborative Data Ecosystems
Collaborative data ecosystems involve partnerships between organizations to share insights. This collaboration enhances competitive advantage by combining diverse datasets for richer analysis.
9. The Rise of Automated Analytics Tools
Automated analytics tools streamline the analysis process. Organizations utilize these tools for rapid insights without requiring extensive data science expertise.
10. Emphasis on Ethical AI Practices
Ethical AI practices play a vital role. Organizations focus on responsible AI use, ensuring that algorithms operate fairly and without bias in data analysis.
These trends highlight the dynamic nature of data and analytics, positioning organizations to harness data effectively in the years to come.
Business Growth
A robust data and analytics framework is essential for organizations aiming to thrive in a competitive landscape. By effectively managing data governance, architecture, quality, and analytics methodologies, companies can transform raw data into valuable insights. Addressing the challenges associated with implementation is crucial for maximizing the framework’s benefits.
As trends like AI, real-time analytics, and data democratization continue to evolve, organizations must remain agile and proactive. Embracing these advancements not only enhances operational efficiency but also positions businesses to make informed decisions that drive growth and innovation. The future of data analytics is bright, and those who adapt will undoubtedly reap the rewards.
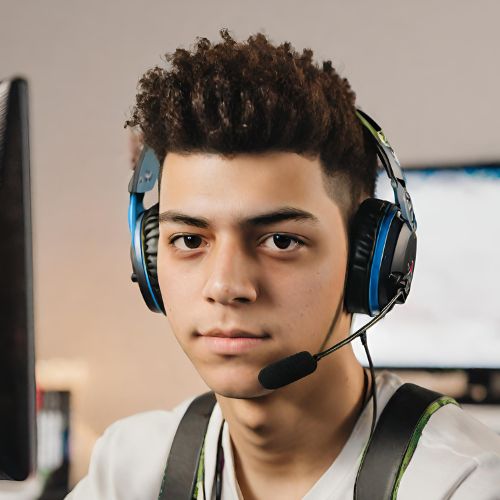